I presented a paper at SAS Global Forum - Orchestrating analytical workloads on Kubernetes powered by GKE on GCP. Link to my paper: SAS on Kubernetes and my presentation is: https://www.slideshare.net/SumanthYamala/sas-on-kubernetes
At Core Compete we enable analytic workloads on containers. SAS on Kubernetes and SAS on Containers articulates our approach.
Describes cloud data warehousing. Processes involved in building a cloud data warehousing - data extraction, data validation, building data pipelines, orchestration engines, monitoring of data pipelines.
Saturday, May 4, 2019
Saturday, January 19, 2019
Idempotency in data pipelines
Any data pipeline will experience a failure and there is no exception. It is how a data pipeline handles these failures and gracefully recovers, differentiates a well designed data pipeline from one that is not. Many applications in the cloud need to be designed to behave in a predictable way. Idempotency in data pipelines will be the focus of this blog.
Idempotence according to Wikipedia is the property of certain operations in mathematics and computer science whereby they can be applied multiple times without changing the result beyond the initial application.
Data pipelines are a sequence of steps to transform and in some cases migrate data. There are applications in the cloud marketplace that help us create these data pipelines - Airflow, AWS Glue, GCP Data Flow, Azure data factory to name a few. Data pipelines involved integration across disparate systems, could involve processing engines like EMR or DataProc, with the purpose to transform and move data. These data pipelines have multiple points of failure and It becomes imperative to plan for failures to ensure data integrity is maintained.
The options to commit/rollback on the entire pipeline never exists. Almost all big data systems do not support multi-statement transactions. Ideal way to handle the recovery from failures in a data pipeline is by enforcing idempotent behavior, i.e. the pipeline can be called with the same set of parameters any number of times, and the output will still be the same. Many of the data orchestration applications that run the pipelines, nudge us to design in an idempotent way, but it is not guaranteed. It needs to be captured by the developer designing these data pipelines.
Points of Failures:
- The pipeline failed before it started
- It failed half way
- The pipeline completed but it failed to return a success code
A data pipeline's design needs to accommodate these failures and handle them gracefully and should self-heal. Having a manual intervention to solve these problems increases the cost to support the application and not sustainable. In many cases asking the question of what if helps us think through the problem.
We will go over two scenarios. Scenario 1 highlights issues even when the flow is idempotent. Scenario 2 identifies how a minor variation in design can make the design idempotent.
Scenario 1:
Lets take a simple pipeline which looks like the following
- Restful data API which extract data from third party data source (daily frequency)
- Insert data from REST API into Cloud Storage (GCS)
- Read data from GCS and apply spark transforms
- load transformed data to Big Query (BQ)
There are multiple approaches to ensure the design of a pipeline is idempotent. One needs to consider processing time, volume of data and most importantly the properties of the underlying stroage sub-system.
Approach 1:
1) GET data from API
2) Put data on GCS with a naming convention gs://inbound_data/data_<date>.csv
3) Truncate partition and load data into BQ partition
In this approach when all things work as planned, there are no issues. What if step 3 fails after truncate? the systems mostly a Tableau/Looker querying BQ will not have any data until we re-run the entire flow. The sequence of operations matters - remember there is no transaction across statements.
1) GET data from API
2) Put data on GCS with a naming convention gs://inbound_data/data_<date>.csv
3) Truncate partition and load data into BQ partition
In this approach when all things work as planned, there are no issues. What if step 3 fails after truncate? the systems mostly a Tableau/Looker querying BQ will not have any data until we re-run the entire flow. The sequence of operations matters - remember there is no transaction across statements.
Approach 2:
1) GET data from API
2) Put data on GCS with a naming convention gs://inbound/data_<date>.csv
3) Apply spark transforms on input gs://inbound/data_<date>.csv
4) Overwrite data of the partition in Big Query
Both the approaches are idempotent, but approach 1 could lead us to a situation where one application is down until we re-run the entire flow again to fix the data.
Both the approaches are idempotent, but approach 1 could lead us to a situation where one application is down until we re-run the entire flow again to fix the data.
REST API idempotency:
Behavior of third party REST API's vary. One needs to understand if the REST API's are implemented in an idempotent way (not the case in most scenarios). In most cases one needs to study the API and identify the API parameters that governs the data being provided by the API. Test and validate. No silver bullet here.
GCS write idempotency:
As you can see, we use the characteristics of object storage to our advantage, the way it treats an object writes as transactional,by coming up with a naming strategy. The step of writing the file, will merely overwrite the existing file, but not creating a new file.
Spark transforms idempotency:
Spark transforms produces a predictable and deterministic output, provided the input is the same. GCS idempotent behavior ensures the input is the same.
BigQuery write idempotency:
Big Query lets us overwrite the partitions, assuming the partition key is based on the <date> column.
Scenario 2:
Intraday flows of reading data from an OLTP system and put it into HDFS every n minutes. Consider this pipeline.
Approach 1 ( not idempotent):
1) Read data from OLTP database using Spark JDBC where timestamp gt. <lastTimeIRan>
2) Apply Spark transform
3) Write to HDFS
4) Update variable <lastTimeIRan>
Approach 2 (idempotent):
1) Read from HDFS identify maxTimeStamp in data
2) Read data from OLTP database using Spark JDBC where timestamp gt. > maxTimeStamp
3) Write to HDFS
The second approach is idempotent, because you can run it any number of times and the system can break down at any step, the data integrity is guaranteed.
In the first approach however if there is a failure between step 3 and step 4 ( the variable <lastTimeIRan> never got updated) , the next time it runs one will end up with duplicate records and the data gets corrupted resulting in downstream data to be corrupted as well. It only requires one bad design to corrupt your entire downstream data pipeline and the analytics or business intelligence that comes out of it.
Idempotent design helps:
1) Operational stability of the solution
2) Building a automated system
3) Ensuring data integrity
4) A good nights sleep!
Summary:
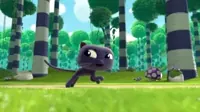
At CoreCompete we build idempotent and reliable data pipelines going through a check list.
Subscribe to:
Posts (Atom)